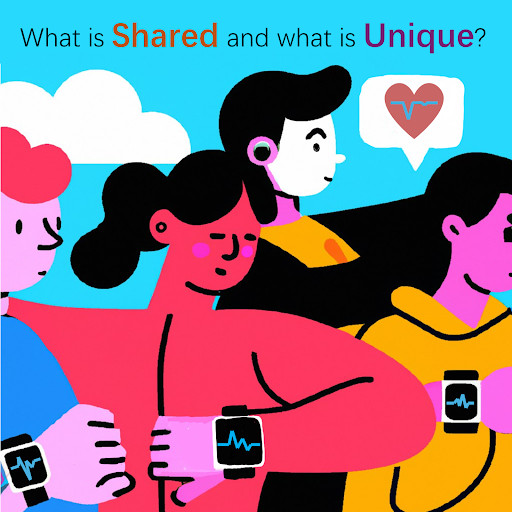
New statistical tool to distinguish shared and unique features in data from different sources
Personalized PCA method overcomes the challenges of heterogeneous data analysis
Personalized PCA method overcomes the challenges of heterogeneous data analysis
When facing a daunting dataset, Principal Component Analysis (PCA), known as PCA, can help distill complexity by finding a few meaningful features that explain the most significant proportion of the data variance.
However, PCA comes with the underlying assumption that all data sources are homogeneous.
The growth in Internet of Things connectivity poses a challenge as the data collected by “clients,” like patients, connected vehicles, sensors, hospitals or cameras, are incredibly heterogeneous. As this increasing array of technologies from smartwatches to manufacturing tools collect monitoring data, a new analytical tool is needed to disentangle heterogeneous data and characterize what is shared and unique across increasingly complex data from multiple sources.
“Identifying meaningful commonalities among these devices poses a significant challenge. Despite extensive research, we found no existing method that can provably extract both interpretable and identifiable shared and unique features from different datasets,” said Raed Al Kontar, an assistant professor of industrial and operations engineering.
To tackle this challenge, University of Michigan researchers Naichen Shi and Raed Al Kontar developed a new “personalized PCA,” or PerPCA, method to decouple the shared and unique components from heterogeneous data. The results are published in the Journal of Machine Learning Research.
“The personalized PCA method leverages low-rank representation learning techniques to accurately identify both shared and unique components with good statistical guarantees,” said Shi, first author of the paper and a doctoral student of industrial and operations engineering.
“As a simple method that can effectively identify shared and unique features, we envision personalized PCA will be helpful in fields including genetics, image signal processing and even large language models.”
Further increasing its utility, the method can be implemented in a fully federated and distributed manner, meaning that learning can be distributed across different clients, and raw data does not need to be shared; only the shared (and not unique) features are communicated across the clients.
“This can enhance data privacy and save communication and storage costs,” said Al Kontar.
With personalized PCA, different clients can collaboratively build strong statistical models despite the considerable differences in their data. The extracted shared and unique features encode rich information for downstream analytics, including clustering, classification or anomaly detection.
The researchers demonstrated the method’s capabilities by effectively extracting key topics from 13 different data sets of U.S. presidential debate transcriptions from 1960 to 2020. They were able to discern shared and unique debate topics and keywords.
Personalized PCA leverages linear features that are readily interpretable by practitioners, further enhancing its use in new applications.
This research is supported by Raed Al Kontar’s National Science Foundation (NSF) CAREER Grant 2144147.
Full citation: “Personalized PCA: Decoupling shared and unique features,” Naichen Shi and Raed Al Kontar, Journal of Machine Learning Research (2024). DOI: 10.48550/arXiv.2207.08041
Written by Patricia DeLacey, University of Michigan College of Engineering